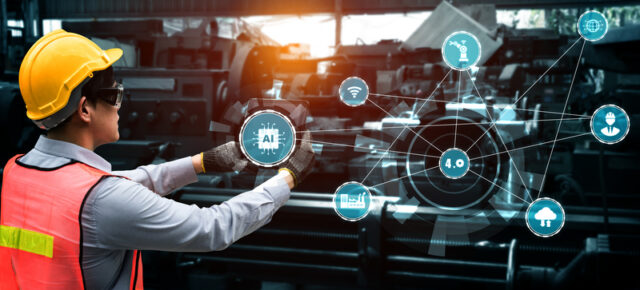
Generative AI is the tech buzzword of the decade — and the money has followed obediently. In 2025, American Big Tech firms alone announced an investment of $300bn in AI infrastructure. A year earlier, global venture capital investment into AI startups reached approximately $97 billion.
One sector that runs the risk of falling behind is construction. ONS Business Insights report only 12 percent of UK construction businesses use AI, which likely reflects (and contributes to) a more skeptical view of AI in the industry. Compared with UK employees across other industries, 11 percent fewer construction employees are excited by the prospect of AI in the workplace and 34 percent of construction workers are worried about the technology.
While cultural barriers may have slowed AI adoption, there are also two major technological challenges that have previously held back AI’s potential for the sector, and therefore likely reduced its use. This year will be the year construction overcomes these challenges and AI becomes a true asset.
New solutions to historic barriers
Firstly, much of the initial attention in the development of Large Language Models (LLMs), the standard foundation for most machine learning protocols, has gone into text-based LLMs.
However, text-based AI alone is of limited use to an industry that revolves around three-dimensional, real-life buildings and sites and which is characterized by huge amounts of often unstructured data. Up until recently, LLMs couldn’t accurately reflect the sheer number of variables on a construction site like dirt and bedrock being different colors, or all the differences in textures and densities. So for effective outputs, the data being input would need to reflect this.
Now, however, AI is going multimodal — and gaining the ability to interpret and analyze the three-dimensional world via pictures, videos, blueprints, and digital scans. This is a gamechanger for the industry. For one example, AI can be used to monitor a live video feed of a construction site to spot safety hazards. For another, it can analyze detailed 3D scans of data centers as they are built — to make sure structural elements of the building don’t interfere with soon-to-be installed electronic IT infrastructure, such as cable ducts.
While multimodality increases the complexity and amount of data needed, this issue is being overcome by specialization in the LLMs — by the variety of foundation models across language, sound, vision, and other modalities, which is reducing the amount of data needed and minimizing the difficulty in creating balance. This also helps to keep development costs down.
Thirdly — and this has been a historic drawback for AI in all scenarios — truly agentic AI hasn’t been possible before today. By this we mean systems that are independent, with access to solutions and therefore impacting outcomes. AI agents take the technology from something that augments a human’s skillset and workload to something that can handle tasks from start to finish.
The key to effective agentic AI has been planning, iteration and reflection, where the AI can learn from previous mistakes for future tasks, leading to continuous improvement. This has been achieved through specific and recent innovations, such as Hierarchical Reinforcement Learning (HRL) — when goals are broken down into sub-goals to improve accuracy — and cognitive architecture inspired by human thinking.
Again, ensuring these agents are accurate and effective — an even bigger concern when they can independently cause outcomes — is helped by creating industry-native environments for these agents to operate in.
Overdue and just in time
Together, multimodal, industry-native, agentic AI solutions have the capability to radically transform the construction site — and we have the capabilities to put these solutions onto sites now. This will create the groundwork for future autonomy in digital and mechanical systems, reducing the variety of skills and number of workers needed on a project. While construction is not the only sector set to benefit from AI and autonomy, it’s arguably more in need of these technologies, due to the structural labor supply issues the industry faces.
Adoption doesn’t have to be all or nothing. Teams can start with a single agent, assess the impact, and expand from there. By layering in AI capabilities gradually, they can build on what works and adapt as they go, creating a scalable path towards a more intelligent, responsive workflow.
Image Credit: BiancoBlue / Dreamstime.com
Karoliina Torttila is Director of AI, Trimble.